Macroeconomy Overview
We are flooded with news and commentary about the macroeconomy. To comprehend it, we must understand the significance of GDP, Inflation, and Unemployment. Identify their 'normal' levels, and examine how they interconnect. Although these levels and relationships are not purely mechanical, they offer a crucial foundation for understanding government actions in a macroeconomy.
GDP
What is GDP - The Circular Flow Diagram
Understanding economic policymaking requires us to start with the essential indicators and primary data that illustrate the dynamics of a macroeconomy. It is important to remember that a country's economy, or macroeconomy, is dynamic, vibrant, and highly complex. It is constantly changing and unstable, so we will focus in these initial minutes on three indicators that you have likely studied. However, to ensure that we comprehend these indicators and their interrelationships, we need to discuss what actions the government should take when assessing the economy and attempting to address any issues. We will begin with what is known as the circular flow diagram to gain an understanding of GDP. Additionally, we will observe the dynamics of a macroeconomy. The first illustration we will create here depicts a very simple economy. There are only households on one side. There are businesses on the other side. There is a relationship between the two, where households purchase goods and services from businesses. The businesses subsequently provide income to households in the form of wages, interest, rent, or other payments.
 |
Picture 1 |
The full circular flow model comprises five sectors:
1. Household sector
2. Firm sector
3. Government sector
4. Foreign sector
5. Financial sector.
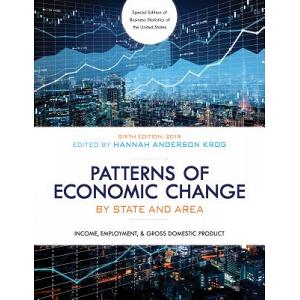
What households purchase from businesses is referred to as consumption, which constitutes the largest component of GDP. The items that people typically purchase for their daily living are quite stable and do not tend to fluctuate significantly over time. If you examine this diagram and consider the bottom arrow, which represents expenditures in the economy, you will notice that it has been primarily driven by consumption. The relationship between the top arrow and the bottom arrow, which represents income in the economy, shows that they are likely to be equal. In other words, if households are consuming 100, businesses will have 100 available to distribute back to households in the form of profits, wages, interest, rent, and other payments. However, if households receive 100 and then begin to consume only 90, businesses will generate only 90 in income during the next cycle. As a result, the entire economy will decline. In other words, the concept is that the bottom arrows represent spending, and the amount spent will influence the top arrows, which represent income. The two will likely move toward equality. It is essential for us to remember this, as it is dynamic. The bottom section influences the top section.
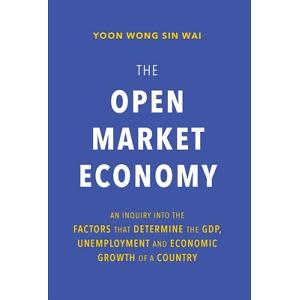
Therefore, after individuals receive their income, we observe that they engage in consumption. However, another option for them regarding their income is that they may choose to save it. We have an arrow pointing out from this point, which represents savings. It flows into the financial sector, represented by the blue circular in the middle. Once it enters the financial sector, it may return to spending as an investment. It is important to recognize that one group of people is responsible for saving, while another group is responsible for investing, so there is no inherent reason for these two groups to be equal. To put it differently, you may be saving money with your future in mind. we are considering how to fund our children's university education, planning for pension, and saving for a house we want to purchase. Meanwhile, investors may borrow the money we have saved. They wish to construct a new building because they believe the future will be very promising. Alternatively, they may choose not to borrow the money you have saved because they believe the future does not look promising, which is the same reason you want to save a significant amount. Therefore, it is possible that savings may exceed investment. Alternatively, investment may exceed savings. If this occurs, would you like to take a moment to consider the impact on the economy. Imagine that there is 100 in the top flow, representing the income, that households receive. They spend 90 and save 10. Therefore, 10 enters the financial system. Now imagine that investors approach the financial system to borrow just five of those ten. We have ten going out and only five coming in, which will lead to a decline in the economy. In fact, in economic terms, we refer to 'injections' as money that flows out of the income stream and contributes to expenditure.
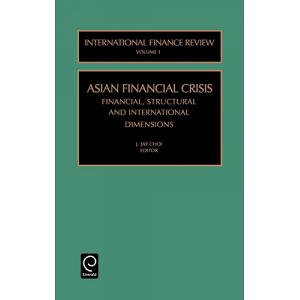
Next, we have what we refer to as 'leakages,' which are funds that exit the income stream. In the picture 1, we are simply illustrating that a flow represents savings. It was generated, it entered households' finances, and they removed it from the income stream. The injection refers to investment. This is money that is outside the system; it resides in the financial sector, and investors retrieve it and bring it back in. So that's an injection. If you consider this, you'll realize that the economy will remain stable when injections equal leakages. Now, if leakages—savings in this case—are greater than injections, which represent investment, it becomes clear that the economy will decline. We withdrew 10, but only 5 came back in. The economy will face a decline. In this regard, it is important to consider the financial crisis. What occurred when the financial crisis began? People became very fearful when they realized that their homes were not worth what they had believed, that their mortgage was much larger than they had anticipated in relation to the value of their home and that they might lose their job. They began to save more, leading to an increase in leakages.
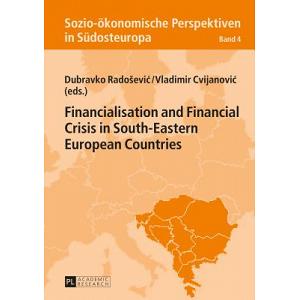
Simultaneously, investors assessed the future and thought, this doesn't look promising. The investors don't want to construct that new factory because they are uncertain whether they will sell what it produces next year. As a result, they began to invest less. Consequently, leakages increased, injections decreased, and the economy declined sharply which indicates the beginning of the financial crisis.
Now, there are several other factors that affect the economy. To briefly summarize, in addition to saving some of our money, we also pay taxes with a portion of it. In fact, we pay taxes at every point in this circular flow. We pay taxes when we receive our income, while we are spending our money, and businesses also pay taxes. Various types of taxes are collected, and these funds flow into the public sector, of course. The public sector then reinvests the money, either through government spending or in the form of transfer payments to individuals, such as unemployment benefits, pensions, and other assistance.
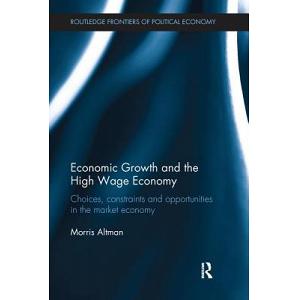
Thus, we observe a new leakage in the form of taxes and a new injection represented by government spending. Once again, when we combine all these factors, we see that all leakages must equal all injections for the economy to remain stable. If all leakages exceed all injections, meaning that savings and taxes combined are removing more from the economy than government spending and investment are contributing, the economy will decline. If all injections exceed all leakages, meaning that investment and government spending combined is greater, they are contributing more to the economy than savings, and taxes are withdrawn. As a result, the economy will grow.
Returning to the financial crisis, we observed that during the initial phase, people started to save more and invest less. Larger leakages and smaller injections. What steps can the government take to address this issue? This is our initial perspective on the government's role. In what ways can the government reduce this impact?
By increasing spending and reducing taxes. This exact approach was taken by the U.S. government immediately following the crisis. A larger injection is needed to compensate for the reduced investment, along with a smaller leakage to balance out the increased savings. This should have done the trick.
The U.S. government responded to the crisis by boosting spending and lowering taxes. This same strategy was implemented right after the crisis. To counteract the decline in investment, this indicates that there is a reduction in the amount of money being invested, which is seen as a problem that needs to be addressed. So, a greater financial inflow is necessary, this means that more money needs to be brought into the system or economy to make up for the reduced investment. This is to balance the rise in savings, this implies that there is an increase in savings, which might be contributing to the decline in investment.
There is one more factor to consider in the economy to fully understand the overall picture of GDP, which is our trade with foreign countries. So, we have a foreign sector involved, and when we import from them, some of our income flows out to foreign countries. This represents a leakage. And we export to them, which transfers some of their income into our expenditure stream; that represents an injection.
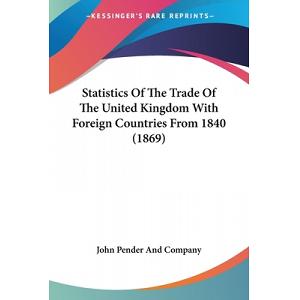
So, if you examine this situation, you can see that one way for countries to emerge from this crisis would be for the new injection we've introduced—exports—to be significantly larger compared to the new leakage we've added, which is imports. If a country is able to export significantly more than it imports, it can make up for the additional savings that occurred due to the financial crisis, as well as the reduced investment that resulted from it. Alternatively, consider a country like Spain, which experienced rising savings, declining investment, decreasing government spending, and increasing taxes. Thus, leakages are significantly high while injections are considerably low. The only way for a country like Spain to make up for the negative impact this has on the economy would be to export significantly more than it imports and strive for growth, which is precisely what it is doing.
This representation of the circular flow aids in our understanding of what GDP is. GDP is essentially the upper portion of this flow in the analysis we're conducting today. This upper section represents all the spending within the economy. If you examine the arrows we've illustrated, you'll see consumption, investment, government spending, and net exports (exports minus imports).
In other words, we have the GDP equation:
GDP=C+I+G+(X−M)
C = Consumption
I = Investment
G = Government Spending
Net Exports = (Exports - Imports)
This can be articulated as follows: GDP is the total market value of all final goods and services produced in the economy within a specific year. We sum up all of our spending on the goods and services produced by the economy to arrive at GDP.
There are two additional indicators we need to discuss. Inflation and unemployment.
We will be particularly interested in inflation. Inflation measures the increase, and occasionally the decrease, in the prices of a basket of goods typically purchased by the average consumer. We generally discuss how much those prices have risen or fallen over the past year. Unemployment is the percentage of the labor force that is actively seeking work but is unable to find it. The percentage of individuals who are either employed or actively seeking employment but are unable to find work despite their efforts. These are the three key indicators we will be discussing: GDP, Inflation, and Unemployment. Next, we will aim to determine the appropriate levels of these indicators and explore how they interact with one another.
Potential GDP as a Reference Point for The Business Cycle
Now that we understand the sources of GDP, we will later discuss the factors that determine the level of GDP in an economy. First, take a moment to examine what GDP growth looks like, and then establish a reference point. This enables us to evaluate whether GDP growth in a specific country at a given time is excessively high, too low, or just right. First, Let's take a look at a screen from Google Public Data. This tool is recommended because it allows you to visualize and compare indicators from different countries over time. We will begin by examining the GDP growth rates of a group of countries over time, specifically from 1960 onward. This represents a significant period. We chose several countries. We have China, Mexico, the United States, Japan, Germany, the United Kingdom, and Greece.
What you see on this screen is the GDP growth rate, which is typically what you will find in news articles discussing GDP trends. They often refer to it simply as the GDP growth rate. This is technically referred to as real GDP growth, a concept you may be familiar with if you have studied economics before. This means that we take the quantity of goods and services produced in, for example, year two, using the prices from year two, and then adjust those prices to reflect the levels from year one.
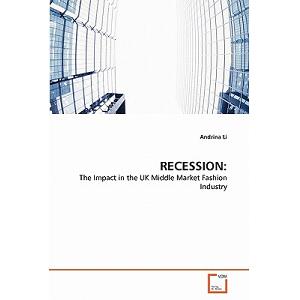
This approach allows us to eliminate inflation from our calculations, enabling us to see the actual increase in the quantity of goods and services produced by the economy. Compared to the previous year, picture 2 removes inflation from all these figures, allowing us to see the actual increase in output from one year to the next across all these countries. For example, in The United States, Looking at the GDP growth, you will notice that it fluctuated around 5% for many years in the 1960s, with some instances falling below that level. In the 1970s, you can observe a recession, during which GDP growth is actually negative. In other words, this refers to a situation where the quantity of goods and services available declines. As we move into the 1980s, we observe another recession. Then, you can observe significant growth rates, again reaching around 5%. Later, as we enter the 1990s, you can see growth rates of around 4%, which is quite strong. Next, you can observe the crisis; in 2009, GDP growth was negative. In other words, the United States is experiencing a recession, and if you examine all the countries in this chart, you'll see that they are all affected as well.
 |
Picture 3 |
The only exception in that year is China, which did not experience a recession according to this data. It is such an outlier; just look at the remarkable growth that China is experiencing. Do you notice that their growth rates occasionally exceed 15%, and at times even surpass 20%? Examining the period since 1990, you can see that the average growth rate was a little over 10% throughout that entire time. For instance, you could consider Mexico and Japan. You can see that their growth rates differ significantly from those of the United States and China.
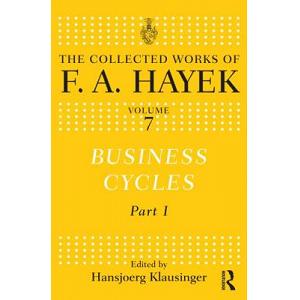
Mexico's growth rate is approximately one and a half times faster than that of the United States, while Japan's growth rate is roughly half that of the United States. Next, let's consider a country like Greece, where you can appreciate the depth of the European Union's economic crisis. You can see that they are still in recession in the end. This raises the question: what is the appropriate rate of GDP growth? Should we aim to grow as quickly as possible? Does the United States aspire to achieve growth rates similar to those of China? Is Japan truly facing difficulties because its growth rate is about half that of the United States? How can we make sense of all this data? To do so, we will introduce a new concept that will serve as our reference point for this course. This is known as potential real GDP growth, which will serve as our target and reference point for analyzing the business cycle.
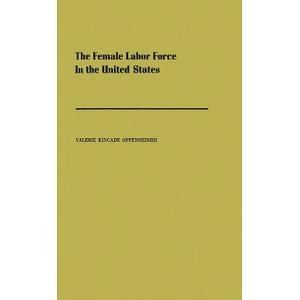
What exactly is potential GDP growth? What does this target mean for the economy? It is calculated by major think tanks and large institutions using a highly technical approach, summing the total investment in the economy and factoring in the growth of the labor force and aspects such as productivity growth.
These are complex models. However, we can calculate it in a straightforward manner that aligns with our objectives for this course by combining two concepts for which data is relatively easy to obtain. One of these factors is productivity growth. Productivity is defined as GDP divided by the number of workers. It reflects the actual output produced by each worker. We measure the growth in productivity, and factors such as education, technological advancements, and management all influence the productivity of each worker, correct? We combine productivity growth with labor force growth. The labor force consists of individuals who are available for work, including those who are currently employed and those actively seeking employment. If demographic growth is robust—such as when people have many children, there is immigration, or the age distribution of the population is leading more individuals to enter the labor force—then we can expect strong growth in the labor force. Conversely, if our population is aging or there is outward migration, we will experience a decline in the labor force. When you combine productivity growth with labor force growth, you obtain a fairly accurate estimate of the economy's potential GDP growth.
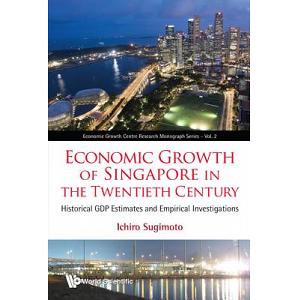
Why is this important to us? The potential growth rate of the economy is the rate at which it can expand over the medium term without resulting in an increase in inflation. In other words, we shouldn't aim to grow as quickly as possible because doing so could lead to an inflation problem. We also don't want to grow too slowly, because, as we all know from experiencing this crisis, it can lead to an unemployment problem. So, what do we aim to achieve? We would like to grow at a rate where inflation remains stable and unemployment is at an acceptable level. What is that rate? It varies significantly between countries.
We presented the two concepts discussed—productivity growth and labor force growth—for the United States over an extended period. By averaging these figures, we derived an estimate of potential GDP growth. You can see that represented by picture 2, which fluctuates but typically remains around 2.5% to 3% per year. That represents the potential growth of the U.S. economy without resulting in an increase in inflation. Maintaining unemployment at a relatively reasonable level over time. Currently, we are working to recover from the unemployment caused by the crisis. However, if we could maintain this growth rate over time, unemployment would decrease to an acceptable level, and inflation would remain stable. This illustrates what potential growth looks like for the United States.
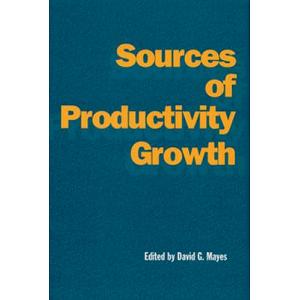
The same approach has been utilized for the 17 countries in the Eurozone. You can see that their productivity growth is actually lower than that of the United States. You can observe that their labor force growth is higher than that of the United States in some years, while in other years it is lower. On average, surprisingly, their labor force growth is higher than that of the U.S. This provides us with a potential growth rate for the countries in the Eurozone; however, this will obviously vary significantly for Germany. For Spain, the figures can vary significantly, but on average, you can see that their potential growth rate is lower than that of the United States. It is approximately two percent. What does this imply? The United States should aim for faster growth than Europe because it has a larger flow of workers and greater productivity growth, allowing it to expand more rapidly without causing higher inflation.
Europe should aim for a growth rate that is somewhat lower, as indicated in this chart, around two percent or slightly below. Japan was included here as well to highlight the contrast. As you can see, Japan's labor force growth has been negative almost every year. This will impact their potential GDP growth and, consequently, the targets for economic policy-making. While their productivity growth is relatively high, the negative labor force growth leads to a lower estimate of potential GDP growth. In this chart, the average was calculated over ten years, and you can see that the potential GDP growth for the Japanese economy is only about one percent. Here, we have a reference point: how much should we grow? Ideally, we should aim to grow at or near our potential. This serves as the foundation for our economic policy-making.
Source:
The Circular Flow Diagram
Coursera